In healthcare, language models are gaining increasing attention due to their ability to automatically process large amounts of unstructured or semi-structured data. “With their emergence, our interest in understanding their capabilities for tasks such as inference with natural language as a data basis is growing,” says scientist Siting Liang, who is advancing the AutoPrompt project in the Interactive Machine Learning research department at DFKI Lower Saxony. According to Liang, Natural Language Inference (NLI) is about determining “whether a statement is consistent with or contradicts the premise”. The AutoPrompt project runs from January to December 2024 and is funded by a grant from Accenture, one of the world’s leading consulting, technology and outsourcing companies.
Siting Liang explains her approach using an example. The starting point is the statement that patients with hemophilia are excluded from a study if certain premises apply, such as an increased risk of bleeding. “This task requires the models to understand the content of the statement, identify and extract relevant information from clinical trial data. The model evaluates whether the evidence supports, contradicts, or is neutral (i.e., neither supports nor contradicts) regarding the statement. Finally, based on the evaluation, the model infers the logical relationship between the statement and the evidence.” she explains.
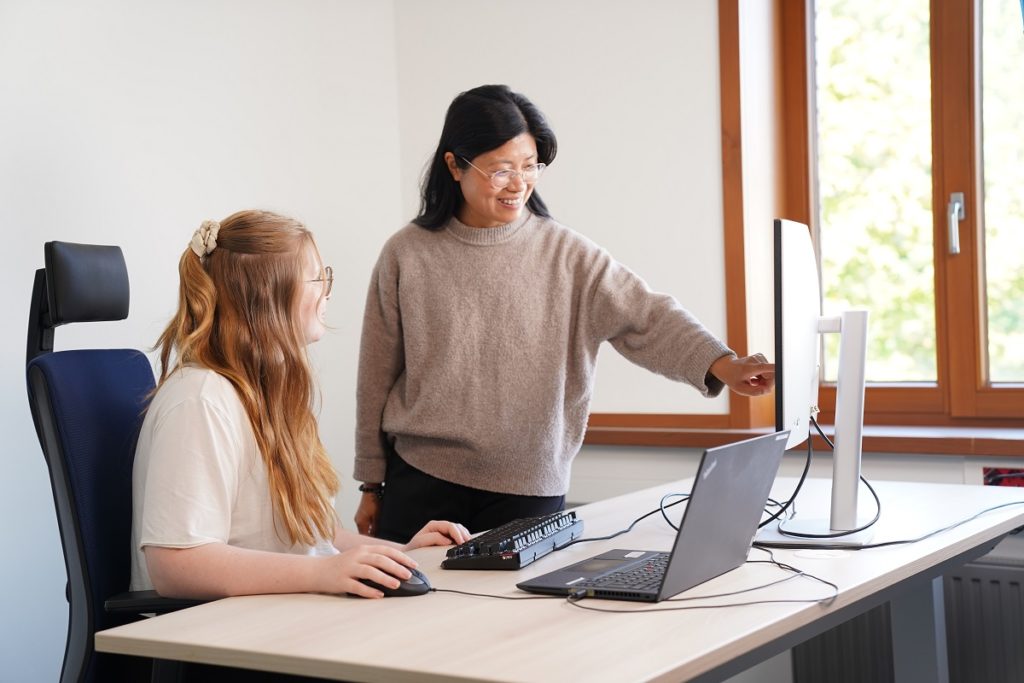
Optimize the prompting
As a first step, the computational linguist wants to optimize the prompting, i.e. the instruction to the chatbot to receive a specific answer. To this end, she researches various strategies such as chain-of-thoughts methods. These involve giving instructions with intermediate steps that follow certain paths and trigger chains of thought. The aim is to elicit a certain degree of reasoning ability from the bot. “ChatGPT may be able to recognize relevant sentences from a context, but drawing precise logical inference requires a deeper understanding of domain knowledge and natural written language,” says Liang.
In a second step, she will evaluate the performance of ChatGPT in NLI tasks using different datasets and suggest improvements. “Our goal is to provide the language models with more domain-specific sources as context,” she says. The goal is to implement the most suitable prompting strategies and a generation framework that enables more efficient access to additional knowledge.
Study with medical students
AI Human Collaboration, i.e. the collaboration between system and human, in this case medical students, plays a major role in the project. To this end, Siting Liang has set up a study within the project, for which she is currently looking for around ten participants. The given statement is that patients diagnosed with a malignant brain tumor are excluded from a primary study if criteria such as chemotherapy apply. The prospective participants are divided into two groups, within which they contribute their knowledge for two hours and make decisions comparing the statement with the clinical trial eligibility data. Group 1 evaluates the decisions of the AI system, and group 2 corrects errors of the system.
“If we want to improve AI systems, we need feedback from humans,” says Siting Liang, who has already worked with medical data in previous projects of the research department. Liang knows that systems can usually analyze medical texts and data very well: “But it is also possible that they hallucinate and give us wrong results. AutoPrompt is supposed to help achieve greater accuracy in the answers.”
Information for interested medical students:
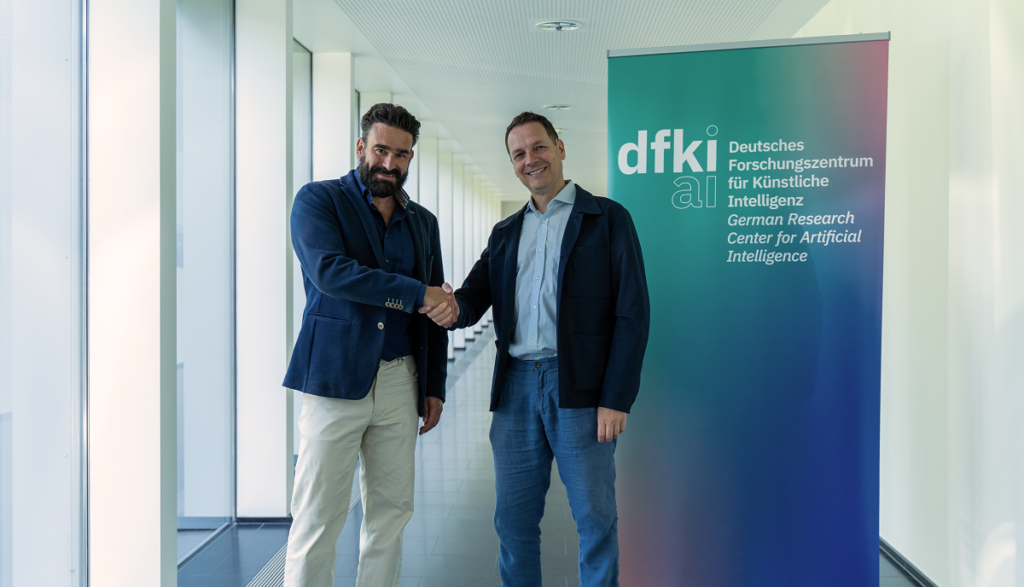
The ‘AutoPrompt’ project was initiated at DFKI thanks to a grant from Accenture Labs. Dr. Bogdan E. Sacaleanu, Principal Director and Global AI Research Lead at Accenture Labs (on the right) and Prof. Dr. Daniel Sonntag, DFKI, have made the collaboration possible.
©DFKI, Simone Wiegand, Jaron Hollax